AUTONEWS
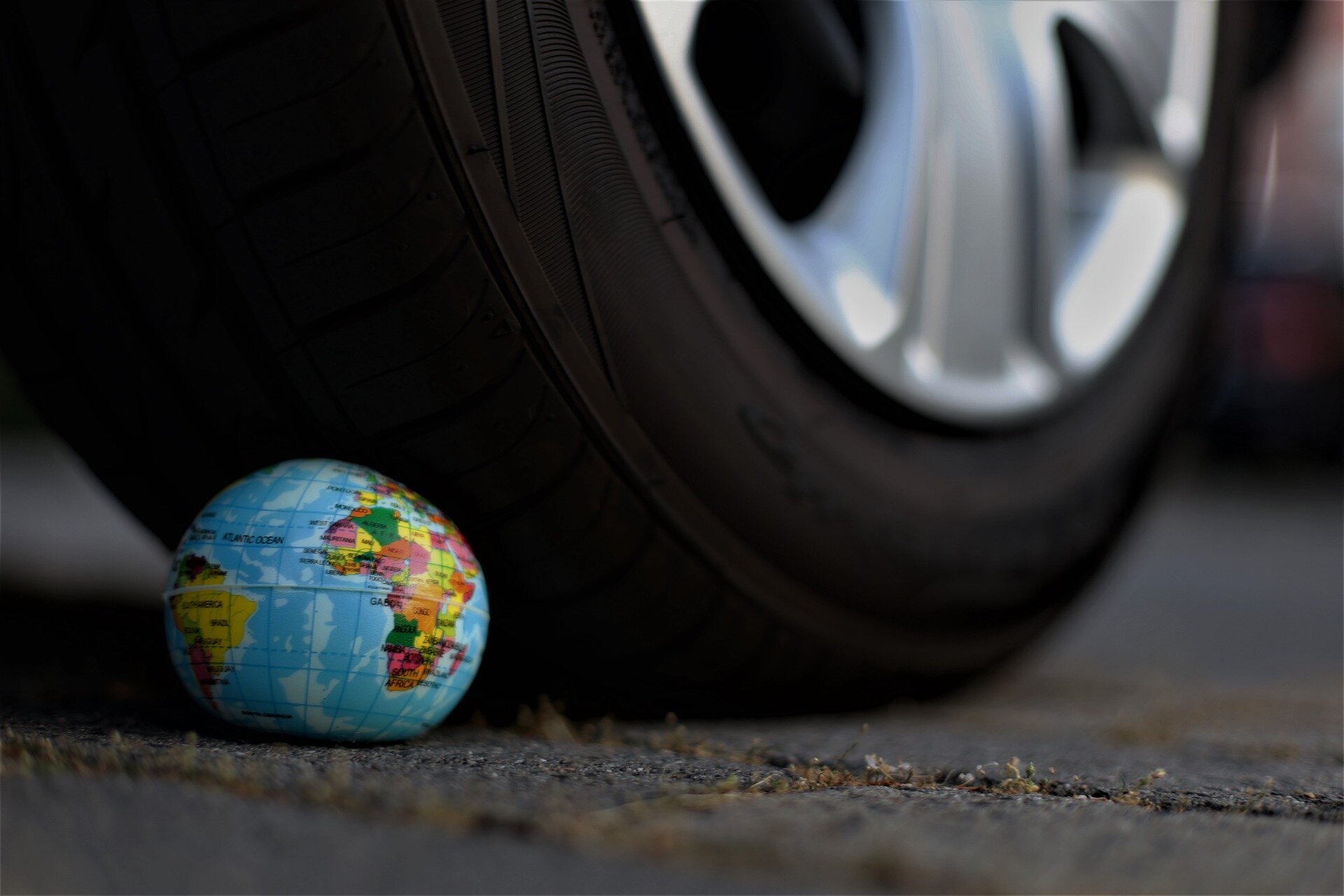
Cutting vehicle emissions and inspections via IoT
Across the U.S., there has been some criticism of the cost and efficacy of emissions inspection and maintenance (I/M) programs administered at the state and county level. In response, Engineering and Public Policy (EPP) Ph.D. student Prithvi Acharya and his advisor, Civil and Environmental Engineering's Scott Matthews, teamed up with EPP's Paul Fischbeck. They have created a new method for identifying over-emitting vehicles using remote data transmission and machine learning that would be both less expensive and more effective than current I/M programs.
Most states in America require passenger vehicles to undergo periodic emissions inspections to preserve air quality by ensuring that a vehicle's exhaust emissions do not exceed standards set at the time the vehicle was manufactured. What some may not know is that the metrics through which emissions are gauged nowadays are usually measured by the car itself through on-board diagnostics (OBD) systems that process all of the vehicle's data. Effectively, these emissions tests are checking whether a vehicle's "check engine light" is on. While over-emitting identified by this system is 87 percent likely to be true, it also has a 50 percent false pass rate of over-emitters when compared to tailpipe testing of actual emissions.
With cars as smart devices increasingly becoming integrated into the Internet of Things (IoT), there's no longer any reason for state and county administrations to force drivers to come in for regular I/M checkups when all the necessary data is stored on their vehicle's OBD. In an attempt to eliminate these unnecessary costs and improve the effectiveness of I/M programs, Acharya, Matthews, and Fischbeck published their recent study in IEEE Transactions on Intelligent Transportation Systems.
Their new method entails sending data directly from the vehicle to a cloud server managed by the state or county within which the driver lives, eliminating the need for them to come in for regular inspections. Instead, the data would be run through machine learning algorithms that identify trends in the data and codes prevalent among over-emitting vehicles. This means that most drivers would never need to report to an inspection site unless their vehicle's data indicates that it's likely over-emitting, at which point they could be contacted to come in for further inspection and maintenance.
Not only has the team's work shown that a significant amount of time and cost could be saved through smarter emissions inspecting programs, but their study has also shown how these methods are more effective. Their model for identifying vehicles likely to be over-emitting was 24 percent more accurate than current OBD systems. This makes it cheaper, less demanding, and more efficient at reducing vehicle emissions.
This study could have major implications for leaders and residents within the 31 states and countless counties across the U.S. where I/M programs are currently in place. As these initiatives face criticism from proponents of both environmental deregulation and fiscal austerity, this team has presented a novel system that promises both significant reductions to cost and demonstrably improved effectiveness in reducing vehicle emissions. Their study may well redefine the testing paradigm for how vehicle emissions are regulated and reduced in America.
Provided by Carnegie Mellon University, Department of Civil and Environmental Engineering
Nenhum comentário:
Postar um comentário